DETECTING PFM-1 LANDMINES
THE PROBLEM
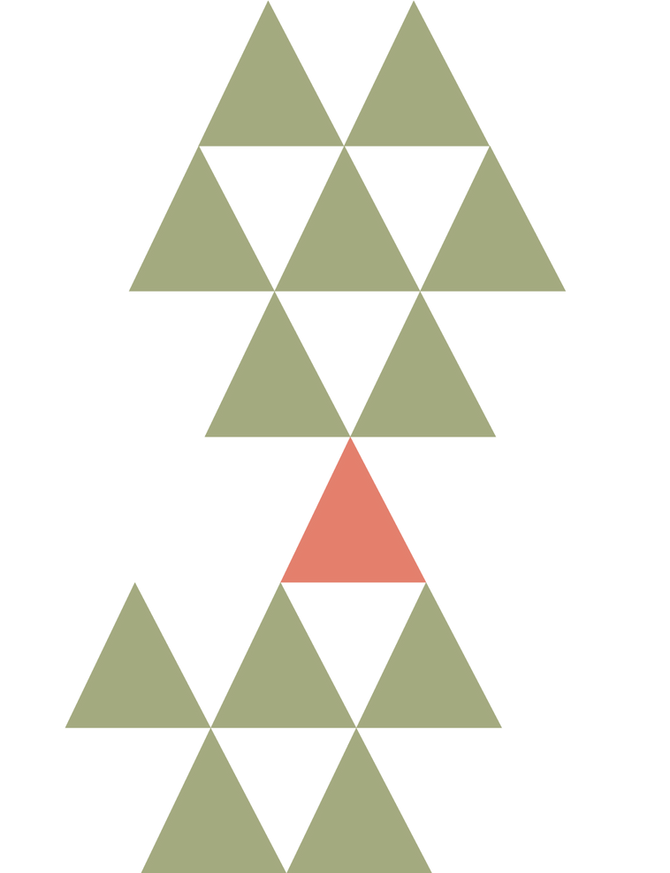
Through synergies in multispectral imaging, thermal imaging, photogrammetry, drone platforms and machine learning, we have devised an innovative way to detect surface-lain PFM-1 anti-personnel landmines. These devices pose a particularly difficult challenge to conventional landmine detection methods such as metal detecting, as the mines are primarily composed of plastic and weigh an average of only 75g. An estimated ten million PFM-1 devices remain scattered throughout Afghanistan as remnants of the ten year Soviet-Afghan war which ended in 1989. These mines remain in isolated locations, frequently out of reach of demining NGOs and act to thwart local economic and social development. The PFM-1s are infamously referred to as “toy mines” as children often mistake them for toys and are injured or killed.

REMOTE SENSING
We have successfully identified landmines using various drones at 10 meters' height through multispectral and thermal infrared imagery with the Parrot Sequoia multispectral sensor, the FLIR Vue Pro R thermal camera, and the DJI RGB camera. Because the mines differ from their host geology in aspects such as reflectance, emissivity, and thermal conductivity, they heat and cool at rates different from their surroundings. In the early morning hours when thermal inertia is greatest, the PFM-1 mines can be detected based on their differential thermal inertia. Because the mine has a predictably different temperature from its background and a distinguishable shape, we have been able to develop a convolutional neural network (CNN) to automate detection of the mines over large areas.
MACHINE LEARNING
The CNN was trained on manually labeled images from over 20 drone flights resulting in thousands of labeled PFM-1 mines. In order to create a robust, generalized machine learning model that can detect real mines in new environments, we collected data from various grass, rubble and sand minefields showing PFM-1s in many different orientations. To date, we have achieved a 92% accuracy in identifying PFM-1 mines in images that the CNN had not previously seen. To identify mines in new environments we input a minefield orthophoto collected from any drone with an attached GPS into our CNN. It analyzes the image and produces a csv file with centimeter scale accurate coordinates where the mines may reside as well as a confidence level metric for each one.

FUTURE WORK & IMPLEMENTATION
This method can be adjusted to detect other types of scatterable antipersonnel mines in future trials to aid demining initiatives. With millions of remnant PFM-1 and similar scatterable plastic mines across post-conflict regions as well as large, undeployed stockpiles of these landmines posing long-term humanitarian and economic threats to impacted communities, our methodology would considerably aid existing and new demining efforts.


Timeline

Watch us present this project at a webinar hosted by the Red Cross and GICHD
Authors:
Baur, J. (Columbia University, New York, NY, United States)
Nikulin, A. (Binghamton University, Binghamton, NY, United States)
de Smet, T. S. (Geological Sciences and Environmental Studies, Binghamton University, Binghamton, NY, United States)
Steinberg, G. (Binghamton University, Binghamton, NY, United States)
Frazer, W. (Yale University/ Binghamton University, Binghamton, NY, United States)
RESEARCH COMPETITION AWARDS

Tech Brief’s Create the Future Design Contest 2018, Aerospace and Defense Category Winner
- Highly competitive award outcompeting NASA and other aerospace and defense organizations from over 800 entries from 60 countries

Finalist in National Geographic's Create the Future: Unlimited Innovation Challenge 2018
- 2nd place overall out of over 5,000 submissions, selected by group of judges and voted by members